Predictive Analytics in the Development of Mobile Apps
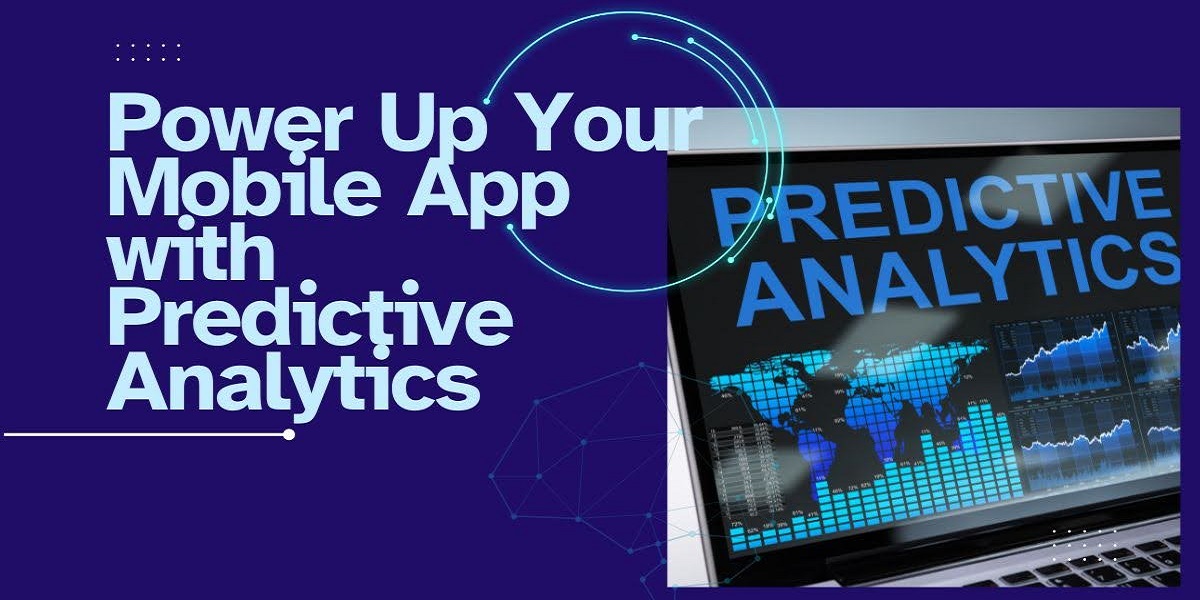
Introduction
To stay competitive, businesses need to be aware of the latest trends. It is now easier to track customer trends using predictive analysis. Businesses can make informed and timely decisions while developing apps and websites; marketing strategies can also be improved depending on data analysis and algorithms.
This article discusses the importance of predictive analysis in businesses to prove them in the future by improving app performance and increasing customer retention for long-term success.
1. Predictive Analytics in App Development
In predictive analysis, the users get significant knowledge about the trends their customers follow through historical data and statistical models. This determines which features the customers will require in future app versions. It gets easier for the developer to spot trends and make predictions. Now gone are the times when developers only used to rely on their assumptions for making apps; they can analyse the data through various tools to optimise their apps. Predictive analysis can:
- Analyse how users behave
- Enhance the user experience
- Enhance the app's functionality
- Analysis of market trends
For instance, Netflix predicts user preferences based on viewing history. Amazon suggests products to users using predictive models.
2. The Importance of Predictive Analytics in Future Apps Development
Predictive analysis has become necessary for businesses to stay ahead in the competitive market. As user needs change with time, through predictive analytics, businessmen can clearly understand the obstacles and opportunities that match customer requirements. Let’s have a look at how businesses are future-proofed by predictive analytics.
Customer Retention
This is a known fact that the success of a business depends on retaining its customers. So, customer retention can lead to long-term success. Companies can better understand the trends their clients are following and adopting, which can help implement desired features in the app. Predictive analytics can also give ideas about when customers are likely to leave the app, which can prevent them from doing so timely. This process helps retain past customers and reduces the cost of acquiring new ones. Let’s take the example of Spotify to make it more clear.
Example: Spotify's predictive analytics give recommendations based on your listening history. User engagement is reduced with personalised recommendations.
b. Personalizing User Experiences
These days, consumers expect brands to provide them with personalised experiences. Based on individual preferences, predictive analytics helps apps customise content and features. Personalisation is one of the greatest methods to keep your users interested and satisfied, as the customers favour it.
Example: The Amazon app uses a user's browsing and purchase history to provide more relevant purchasing results.
c. Improving App Development
By identifying aspects that users are expected to find enjoyable, predictive analytics can direct the creation of apps. Developers may cut costs and time by prioritising features that generate engagement. Better software and a quicker market launch are the outcomes. This is where app-developing companies can benefit.
Example: fitness applications can utilise predictive data to determine which features—like recording exercises and providing individualised fitness plans—users use more frequently.
d. Enhancing Security Protocols
As all businesses are going for cybersecurity, security is a top priority for application developers. Security is even more important for apps that handle sensitive or personal information. Companies can identify fraud or system breaches by analysing previous data using predictive analytics. This proactive strategy strengthens app security and data protection.
Example: PayPal detects fraudulent transactions using predictive analytics. This highlights questionable behaviour before it becomes an issue.
3. Importance of Predictive Analytics in App Creation
Predictive analytics can enhance app security, usability, and usefulness throughout the development cycle. The following fields are greatly benefiting from predictive analytics:
An Analysis of User Behavior
To enhance the features and appearance of an application, it is crucial to know how customers engage with it. For this reason, predictive analytics gives insights about the features customers most want. It also predicts the problems customers face and how they use their apps. Hence, a developer can better create apps satisfying customers’ demands by anticipating their behaviour.
Example: A food delivery service can benefit from predictive analytics by determining which places are getting more weekend orders. With this knowledge, weekend promos and personalised deals can be developed.
Performance Monitoring
Predictive analytics can anticipate performance problems like crashes or sluggish load times. Real-time app metrics analysis enables companies to fix possible problems before they negatively impact users' and other users' experiences. A predictive model should be employed to ensure that apps function properly and live up to user expectations.
Example: Facebook uses predictive analytics to track platform performance and forecast crashes, guaranteeing that problems are fixed early.
Fraud Detection and Prevention
Fraud Detection and Prevention One of the most exciting features of predictive analytics is its ability to detect fraud activity in real time by tracking transaction patterns and detecting abnormalities.
This way, remedial or prevention methods can be adopted beforehand to avoid fraud. Developers need to ensure that their apps are free of scammers and frauds.
Example: Financial apps like Venmo employ predictive analytics to identify suspicious activity and protect consumers from unwanted access.
Optimisation of Marketing Campaigns
Predictive analytics can be a great tool for marketing agencies to improve their campaigns. Businesses can benefit by expanding their customer base through reaching out to a wider audience that can turn into leads.
Also, data analysis can be utilised to anticipate and predict the members of the customer base who are most likely to respond to a specific promotional event. This leads to tailored marketing to increase conversion rates and return on investment (ROI).
Example: Apps that offer e-commerce can use predictive models to identify which users are more likely to abandon their shopping carts and then provide incentives to complete their purchases.
Personalised Recommendations
Personalised suggestions improve the user experience and help engage users. Businesses can customise their products and services based on their customers' preferences by analysing what features, products, or content they like.
Example: YouTube's video recommendation system uses predictive analytics to make video recommendations.
4. Real-World Applications and Case Studies
Several companies have used predictive analytics to improve the functionality of their applications and increase business growth. Below are a few examples:
Uber: Uber utilises predictive analytics to forecast demand in different geographic areas. This app uses historical data to predict future car demand, reducing waiting times and increasing driver availability.
Amazon: Amazon uses predictive analytics in its product recommendation engine. Amazon predicts the items for each customer based on their browsing history and recommends similar or desirable items to increase sales.
Netflix: Netflix is now a widely used app that recommends movies and TV shows based on audience preferences. In this way, it keeps the subscribers engaged and reduces turnover.
Zillow: Zillow uses predictive analytics to calculate the prices of homes. Zillow analyses previous sales to help buyers find the perfect property.
You may observe how predictive analytics can improve app performance, boost user pleasure, and propel business success within these organisations.
5. The Challenges of Implementing Predictive Analysis
There are also some disadvantages to predictive analytics.
Privacy Issues with Data
As a result, data-driven technologies have raised several privacy concerns. Businesses are responsible for processing personal data according to the GDPR and CCPA laws.
The solution: Companies must be transparent and honest with the data they collect and use. To build trust, it is important to have clear privacy rules and data security procedures.
Quality of Data
Predictive analytics can be very effective if the data is of high quality. Incomplete or incorrect data can lead to unreliable predictions. A clean, reliable data set is essential to gaining meaningful insights.
The solution: Regular audits and verification procedures are needed to maintain accuracy.
Technical Expertise
Predictive analytics implementation requires knowledge of data science and machine learning. Small firms find it challenging to hire and train employees.
The solution: Businesses might invest in training programs or collaborate with outside data analytics companies to upskill their current analytics industry teams.
Integration with Existing Systems
Predictive analytics integration with the current app infrastructure can be difficult. Legacy models may be unable to support advanced analytics; thus, they need to be updated or completely redesigned to make room for predictive models.
The solution is to use skilled data engineers to guarantee the smooth integration of predictive analytics into app infrastructure.
6. Optimal Techniques for Applying Predictive Analytics
Following these best practices will help businesses make the most of predictive analytics.
Establish Specific Goals
Before using predictive analytics, businesses must establish certain goals. Whether enhancing user experience or customer retention, a clear objective guarantees the effective application of predictive analytics.
Focus on Data Quality
Ensure the analysis's data is correct, comprehensive, and pertinent. Inaccurate forecasts from poor data harm a company's bottom line.
Test and Refine Models Continuously
Testing and improving predictive models is important because they are always changing. Models must be updated regularly with new data to keep them accurate and current.
Communicate with Users
Transparency when dealing with consumer data is essential. Users should be able to control what data they provide and be informed of how it is used.
Investment in Skilled Teams
Predictive analytics requires the expertise of analysts, data scientists, and machine learning professionals. To ensure the success of your predictions, you must engage qualified individuals or invest in training.
Conclusion
Predictive analytics can be an effective method for ensuring that applications are future-proof. Data-driven insights can help improve app performance, security, user experience, and retention.
To implement predictive analytics, businesses need to hire staff with extensive knowledge and understanding of the data management process. Businesses that use predictive analytics in the app development process might better adjust to market changes while remaining competitive.
Businesses can use predictive analytics to create apps anticipating future needs and meeting user demands. It increases the likelihood that these apps are successful in the long run.