Explained! What are the Real-World Applications of Generative AI?
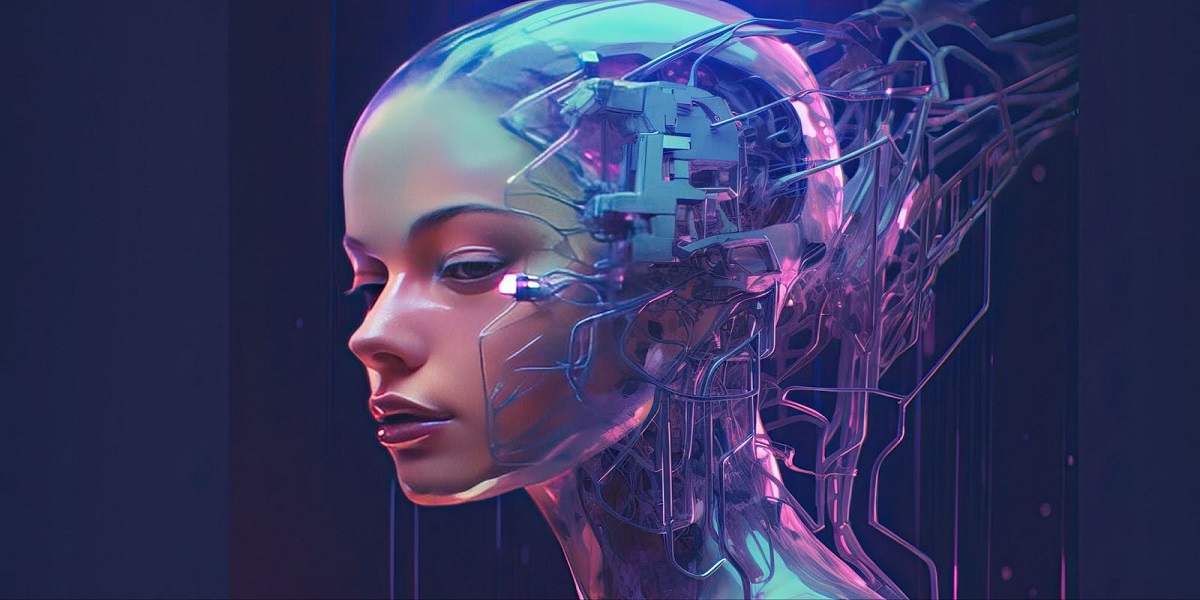
Have you been experiencing how artificial intelligence is surpassing all innovations and suddenly emerging? Witnessing how it has taken charge of every task and conducted them effortlessly and efficiently is commendable. Isn't it? We witnessed AI's revolutionary and game-changing tech advancement, but the launch of generative AI development has shaken the whole world. The way it assists, the way it functions, and how it manages all the tasks that need extra workforce and effort. Build Future AI develops best-in-range Generative AI solutions that drastically reduce business expenditure on multiple resources by automating several tasks and duties, offering a seamless and cost-effective solution for businesses at large.
What is Generative AI?
Generative AI is a robust version of artificial intelligence that employs deep learning models and advanced AI algorithms that can assist in developing high-quality text, images, and other relevant content based on the data used to train the model. Gen AI utilizes the data or all the information that can be accessed to embrace knowledge and deliver the relevant answer when the prompt is given.
Types of Generative AI
Following are the types of generative AI using a wide range of machine learning and deep learning techniques:
1. Transformer Models
Transformer models are the neural network models that understand context by tracking the relationship between sequential data, such as the words in a sentence. They are widely used for natural language processing models to make the machine understand the context of the user’s text input. Famous text generation models like ChatGPT 3 and ChatGPT 4 use transformer-based models to generate highly coherent and contextually appropriate text.
2. Generative Adversarial Networks (GAN)
GANs are used to create new types of media content that show real people doing different things. It uses 2 neural networks to do so; the first is the generator, and the other is the discriminator. The generator generates new content and passes it to the discriminator, who then identifies whether it is fake or real. With every use, the generator improves in creating new fake content that seems real, and the discriminator learns to identify the difference between fake and real content. Creating such content is also known as “deepfake,” and this ability of AI holds immense potential in the fields of creativity, art, and content creation.
3. Variational Autoencoders (VAEs)
Variational autoencoders are another type of AI that uses principles of statistical inference to generate new content from a dataset. This works by inputting the data into a lower dimensional space to compress its representation and then decoding this compressed data to generate new information.
What can Generative AI do?
Generative AI can expand the reach of artificial intelligence and promote its adoption even to people and firms that do not have data science expertise. ChatGPT is the biggest example of how generative AI models allow laymen to dynamically utilize AI in their day-to-day lives. Moreover, Adopting a generative model for a specific task can be done with minimal data or examples using APIs or prompt engineering, though significant customization still needs expertise. Below given are the three categories vastly supported by generated AI:
Content and Ideas Generation
Be it text, speech, art, images, videos, voice, and ideas, generative AI can be used by commoners to create content according to their requirements in a fraction of seconds.
Improving Efficiency
By reducing repetitive and manual tasks like email writing, coding, or summarizing large documents for users and companies, AI boosts operational efficiency and allows users to focus on more important and creative tasks that are more human-dependent.
Personalizing User Experience
Generative AI creates content and information personalized to the needs, interests, and habits of the audience. This can further enhance the user experience and help in tailored digital marketing, which will boost sales.
The Real-World Use Case of Generative AI
Following are the areas utilizing the potential of Generative AI explained in detail:
Communications
GenAI helps companies or businesses establish an effective communication channel that can assist clients around the clock. Companies employ Gen AI-powered chatbots and virtual assistants, transforming the customer service ecosystem's communication landscape. Gen AI enables personalized content creation, keeps marketing communication highly precise, and provides a tailored solution for customer support interactivity.
Energy
Artificial intelligence and data analysis are fundamental to each other, so the energy sector needs GenAI for its efficient asset performance management. It reduces complexity, manages high-frequency data, reduces unplanned downtime, maximizes downtime, and drives high-value automation. Also, GenAI will assist the energy sector in analyzing the dynamics of wind, speed, solar irradiance, and other energy resources to check the certainty and uncertainty of profits and efficiency in the renewable energy market.
Financial Services
Generative AI plays a significant role in helping financial businesses automate, streamline, and make operational workflows more efficient. Using GenAI, businesses can revolutionize their investment strategies by analyzing customer data, market trends, and economic indicators and launching personalized recommendations and investment plans considering individual risk profiles and financial aims.
Gen AI also assists financial institutions in detecting fraud and preventing it in the financial sector. It also analyzes transaction patterns, customer behavior, and data important for fraudulent activities.
Healthcare and Life Sciences
In healthcare, using vast data sets and research plays a significant role; it requires an in-depth analysis to make a clinical decision that benefits the patient's health concern and maximizes the treatment's proficiency.
As Gen AI works on well-trained models, it assists healthcare professionals in their accelerated drug discovery while managing long, detailed databases into simpler formats. With the help of GenAI, healthcare has become more personalized and effective for treatments. It records the patient's medical history and generates a tailored treatment plan, eliminating the risk of adverse reactions.
Manufacturing
Generative AI helps manufacturing units streamline production management, increasing efficiency and minimizing operational costs. The employment of AI-powered systems elevates predictive maintenance and forecasts the expected machine error or failure by analyzing their condition beforehand.
The use of AI in manufacturing units automates production and improves quality control. AI models used in Gen AI detect the data and identify the potential lags to make adjustments and optimizations, boosting efficiency and improving overall product quality.
Gen AI suggests predictive maintenance, preventing equipment failure, and analyzing the data history of previous records to identify patterns when the equipment needs immediate maintenance.
Media & Entertainment
Generative AI works more seamlessly and proficiently in the media and entertainment industries. It analyzes all shifting consumer behavior to determine what kind of marketing and content creation will work for marketing and media; it automatically plans and schedules those posts by researching the high conversion time frame.
GenAI enables media and entertainment to streamline their content creation process and create original scripts, articles, song lyrics, and whatever is associated with the content part. With Gen AI, the media industry never fails to revolutionize its personalized content recommendations. It gives results based on the user's preferences and viewing habits to enhance the overall user experience.
Retail and consumer goods
The industry linked to retail and consumer goods can no longer afford the transformative vision of generative AI. It adds personalized product recommendations that consider users' preferences and buying personas. The AI models used in Generative AI analyze the customer's data, purchase history, and regular browning behavior by focusing on certain items they want.
Generative AI supports businesses in inventory management and forecasting by predicting demand, optimizing inventory levels, and automating the refilling of items. Using these insights, the user can determine the alignment between supply and demand dynamics.
Public Sector
Diverse sectors fall under the public sector, such as defense, intelligence, transportation, civil services, healthcare, infrastructure, etc. The public sector implements the advanced application of GenAI to provide safe and effective solutions. It empowered the public sector to develop more informed and effective policies. Analyzing the huge databases, demographic information, economic indicators, and social trends for custom policy recommendations addressing the unique challenges and community needs.
Gen AI's major use in the public sector is its enhanced mission delivery into various government agencies and missions ranging from defense to intelligence for a community. Evaluating the most historical data, real-time information, and other relevant data sources, AI models allow Gen AI to predict potential threats and identify the major risks associated with any policies, suggesting an efficient mitigation plan.
Challenges of Generative AI
Following are the challenges coming in the way of development of Generative AI:
Data Requirements
Generative AI provides users with various kinds of information, including sensitive topics. Therefore, training it with a high-quality dataset is important to ensure its accuracy and reliability. However, the challenge is with the availability of data. Most of the data is either low quality or has biases, missing or incorrect values. Hence, to tackle this challenge, investing in data cleaning and data validation is crucial for building a high-quality Gen-AI model.
Training Complexity
Training Gen-AI models, especially complex models like GANs and transformer-based models, are computationally intensive, expensive, and time-consuming. Therefore, any organization would need to have enough resources and expertise, which can be a challenge for small companies. However, using certain techniques like distributed training (splitting the training tasks across different systems) and transfer learning (where already existing AI models are fine-tuned) can ease the training process.
Controlling the Output
Like any other AI model, hallucinations in AI also exist in gen-AI models, and they may produce faulty, offensive, and undesirable information that can hurt people’s sentiments. Therefore, such incidents can be avoided by controlling the output of the gen-AI model. To deal with this challenge, the AI model must be trained with diverse and representative data. Also, special mechanisms to validate the information produced and check for hate speech and sexual or offensive content should be embedded for public safety.
Ethical Concerns
Ensuring the responsible use of AI is another hurdle that can come up in the way of the positive development of artificial intelligence. GANs produce deep fake videos, or any false content can be used by people to spread misinformation or for fraud and scams. Some other examples are writing counterfeit reviews, news articles, wrong educational articles, and hate speech. Therefore, keeping ethical considerations in check is needed before developing or launching a gen-AI model.
Regulatory Hurdles
The need for proper regulatory guidelines for developing or using gen-AI models is another challenge that the AI sector might face. With the rise of AI, the risk of scams and fraudulent activities is also increasing. Therefore, governments and corporations worldwide must introduce laws and regulations to keep a check on the overall usage of AI.
Lack of Transparency
Generative AI models produce the text or visual response in a fraction of a second, but the source of its information and imagination remains unknown. This makes an AI response less credible and has greater chances of error. Therefore, ensuring the reliability of model-building models with transparency or flow-of-conduct is necessary for gaining the public’s trust in AI.
Cybersecurity
Cybersecurity scams are vast and of different types, and detecting them takes work. There is a greater chance that Gen-AI-produced content is prone to be a part of many cybersecurity scams and frauds. Deep Fake videos, voice messages, and offensive texts are some examples that scammers can use to commit fraud. Therefore, it is important to opt for secure databases and authentication models to keep an eye on AI’s activity.
To tackle all these challenges, any company or business willing to launch its own AI model must choose a reliable AI development company with experience in data cleaning and validation, and providing continuous support and improvements is important. Build Future AI and Blocktech Brew are two of the top firms that you can consider when developing an out-of-box AI solution.
In the end,
As Gen AI continues to expand its potential daily, it offers extensive use cases in diverse industries, using revolutionary technical advancements, developing new opportunities for enterprises, and maintaining existing efficiency.
Industries can seamlessly write their marketing copy using generative AI, develop an effective marketing plan, and more. It has ample use cases for different industries that help businesses elevate efficiency and maximize business potential. If you are also searching for employing Gen AI's solutions in your business, don't think twice; hire a top Generative AI development company as it works seamlessly for all industries.